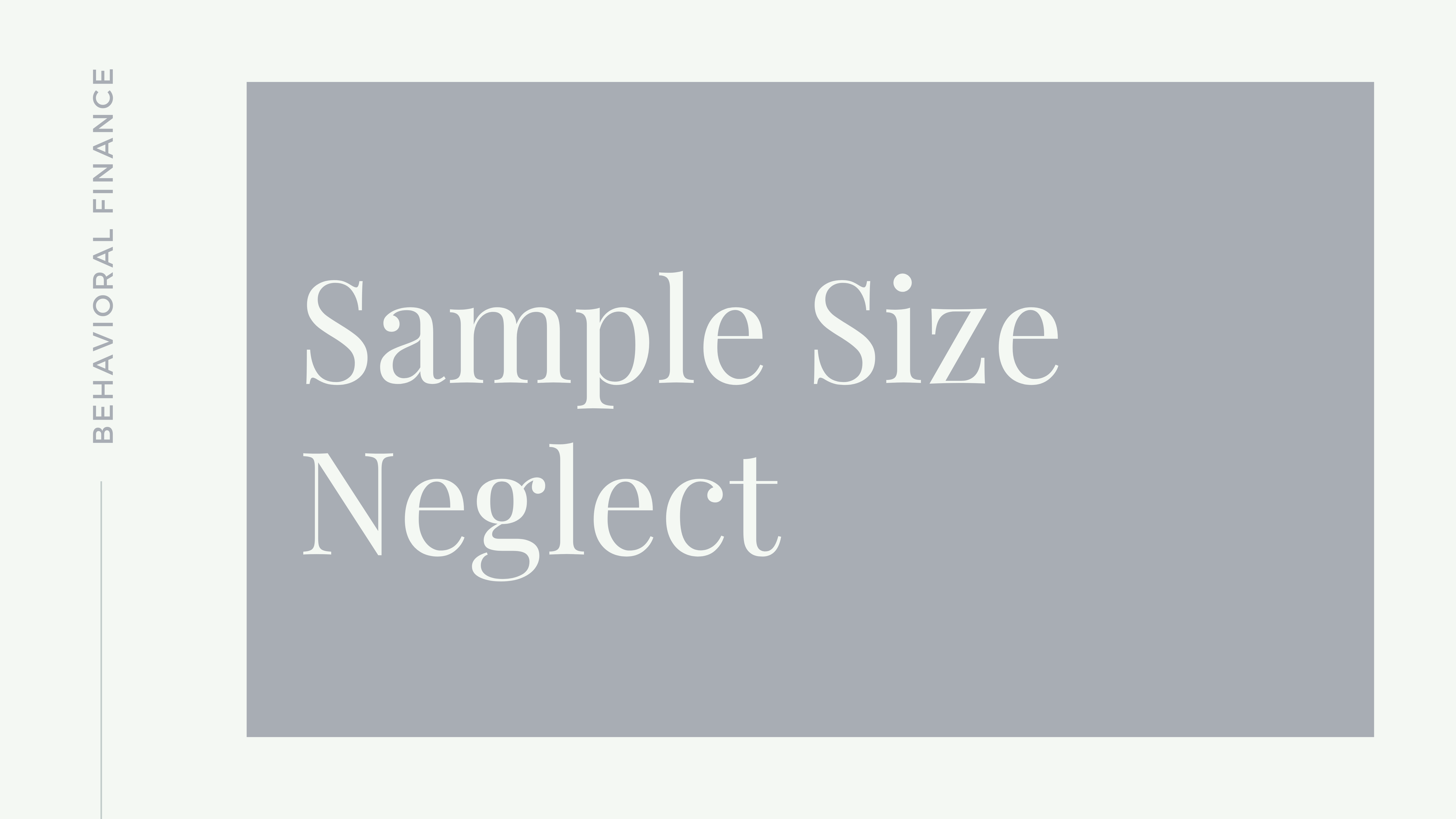
Sample Size Neglect
Sample size neglect is a bias where one evaluates statistical information and arrives at an erroneous conclusion after failing to consider the sample size of the data set. Ultimately, small samples are more likely to contain high degrees of variance amongst data points. If a sample size is not large enough, the data is not necessarily informative, though individuals may be inclined to rely on it and draw conclusions anyhow.
This cognitive bias was first studied and documented by Amos Tversky and Daniel Kahneman who we have covered in previous posts. An example of the bias drawn from their research helps to illustrate the point.
A person is asked to draw from a sample of five balls and finds that four are red and one is green. Another person draws from a sample of 20 balls and finds that 12 are red and eight are green.
Which sample provides better evidence that the balls are predominantly red?
Most people say that the first, smaller sample provides stronger evidence because the ratio of red to green is higher than the larger sample. In reality, the higher ratio is outweighed by the smaller sample size. The sample of 20 provides significantly stronger evidence that the balls are predominately red.
Within the context of personal finance, we often see sample size neglect when individuals evaluate an investment’s past performance. An investor may be presented with their 401k investment options menu along with the 5-year trailing return for all the available investments. In almost all cases, investors will select investments strictly based on the best 5-year return figures. First, they are ignoring the standard disclosure, past returns are not indicative of future results. Moreover, they are overlooking the fact that 5 years is a small sample size in the world of investing. Over such a short period, fund performance could be driven more by broader market conditions or other anomalies, versus superior investment strategy or skill. In this example, returns are a valid data point. However, it is a data point drawn from a small sample and, as a result, should not be relied upon to draw conclusions. One may infer far more about the likelihood of future fund performance by reviewing other data points unrelated to time, such as portfolio turnover, Sharpe ratio, expense ratios, attribution analysis, upcapture/downcapture, investment methodology, etc.
How to avoid sample size neglect
When evaluating financial data, consider how large the data set is and if it is adequate to draw meaningful conclusions. Small data sets will contain high degrees of variance, which can skew data both positively and negatively. If you determine the data set is not sufficiently large, consider alternate data points that could be utilized to provide additional context. Proceed with caution.
This is not a recommendation and is not intended to be taken as a recommendation. This material was prepared for general distribution and is not directed to a specific individual.
LPWM LLC does not provide tax, legal or accounting advice. This material has been prepared for informational purposes only, and is not intended to provide, and should not be relied on for, tax, legal or accounting advice. You should consult your own tax, legal and accounting advisers.